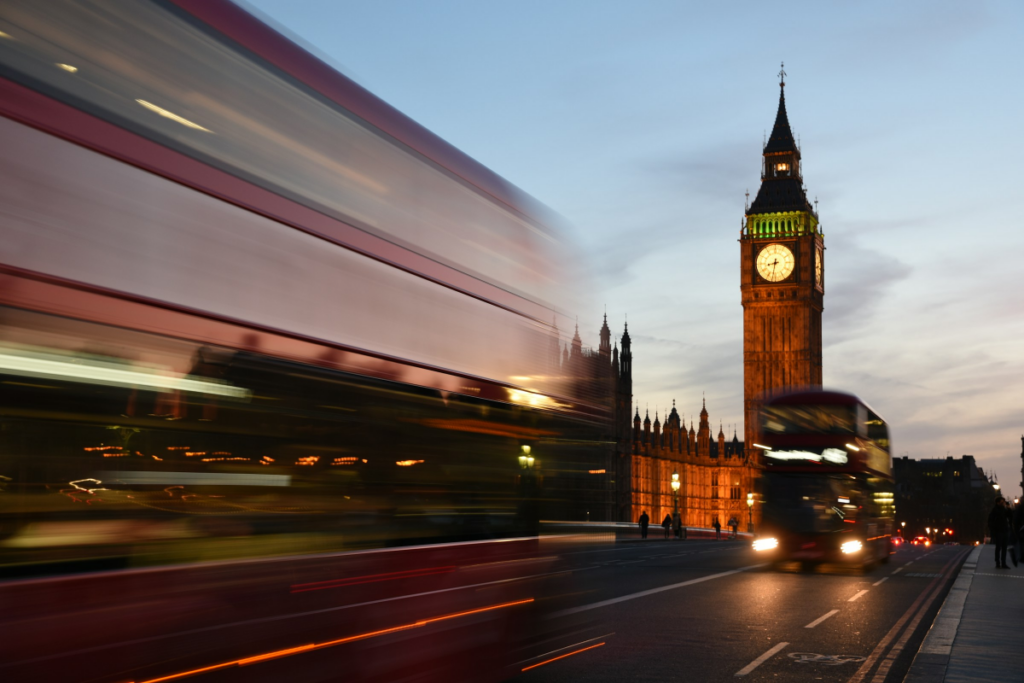
AI was named as 2023’s ‘word of the year’ and over the past 12 months, discussions have only intensified.
The UK is looking to capitalise on the opportunities presented by the latest generation of AI technologies, with one of new Prime Minister Sir Keir Starmer’s early announcements focusing on moving ‘from an analogue, to a digital NHS’ as well as highlighting plans to modernise several other key services. But going beyond the hype, how could AI actually benefit the public sector in the UK , and how do we remove some of the barriers to adoption?
AI in the public sector
Many of the suggested uses for emerging platforms and technologies like AI in the public sector are not particularly ‘glamorous’ (but then, glamour isn’t what the public sector is about, anyway – I think Steve Messer is on the money when he says that at its best, the public sector creates ‘boring magic’). Glamorous or not, their potential efficacy is clear. In a sector driven by data, documentation and administrative tasks, many use cases for AI are immediately obvious.
At a practical level, this means huge arrays of challenging and comprehensive public consultation data, for example, could be analysed with superhuman rapidity. Telemedicine and chatbots could be implemented to support medical appointment scheduling or triage non-emergency cases. In transport, AI could be leveraged to optimise traffic management systems or for smart infrastructure maintenance. It could manage communications processes with the public in issues like taxes and benefits, while automating dozens of processes and improving accountability in public spending.
Experimentation is already underway in the UK government, exploring a wide range of use cases. Importantly, these experiments are multi-faceted, considering things not only from a technical point of view, but also in relation to law, ethics, communications, and public trust, amongst other perspectives. For example, while correspondence with constituents is an obvious use case for automation, what impact might that have on public perceptions of government as remote and detached?
For anyone questioning why AI adoption in the public sector has to date been slow, these broader considerations are part of the answer – but not all. There are a few major blockers preventing progression and further uptake.
Ageing architectures
The first is that there’s still a significant legacy IT estate in the public sector which complicates and impedes the introduction of innovative technologies. These older systems are having a major impact and will constrain the Prime Minister’s ambition to evolve public services, including the NHS. Several key services that play a pivotal role in day-to-day public life are based on platforms that were built pre-millennium, and some are more than four decades old.
While the scale of the legacy challenge is daunting, there are a number of pragmatic strategies government can adopt. For example, we worked with a central government department to improve its citizen-facing services by implementing an event-driven architecture (whereby decoupled systems communicate and react to specific events in real time rather than following a fixed sequence of steps) around its legacy data store. Avoiding the significant expense and time required to replace the legacy system, the solution wrapped a modern architecture around the old one and enabled a shift to data streaming. With this in place, updates to citizen data by the department’s customer-facing teams could be propagated across all systems in real-time – something that was impossible with the legacy system. In this way, departments and public sector bodies can take an incremental and iterative approach to remove obstacles presented by legacy IT.
Furthermore, AI can help governments to implement AI. What do I mean by this is? Well, if a mainframe or a database is decades old, it’s more than likely that the people who originally built it have long since moved on or retired. These systems may still underpin critical services for departments, but no one fully understands how they work, and reverse-engineering them can be a significant, time-consuming challenge; exactly the kind of challenge to which AI is suited. The pattern recognition capabilities of AI and machine learning make them the ideal tools to unearth business logic from mainframes and databases, helping software architects accelerate the migration path from the legacy estate into the cloud.
Data dilemma
One sure-fire approach to improving public services is to break data out of siloes and combine it with other relevant data, both within and between departments. Sharing data is not always second nature to the Civil Service, and while it’s reassuring that the government takes its stewardship of citizen data extremely seriously, unyielding governance can be a blocker.
The pandemic response in the UK showed what was possible. Within two to three weeks of the first lockdown being enforced, GDS, NHS Digital, and what’s now called the Department for Levelling Up, Housing and Communities, had worked together to establish the Clinically Extremely Vulnerable People Service. We were proud to play a role by supporting NHS Digital to create the Shielded Patients List, combining GP records and diverse datasets that were normally only used in trend analysis. While it’s true that special legal provisions were introduced to allow additional data sharing with a ‘COVID-19 purpose’, the pandemic also revealed to civil servants how much data sharing was permitted within existing legislation.
More data sharing will not only improve public services, it’s also a prerequisite for the successful harnessing of AI in government. For machine learning and AI models to fulfil their purpose in carrying out tasks beyond human capabilities, they need to be trained on large amounts of data. Work is already underway in helping departmental teams to break down data siloes and create data pipelines to feed models with the training data they need. And the beauty of it is that it’s a virtuous circle. Once you have improved your data infrastructure to implement AI, AI can help you improve your data infrastructure – extracting, harmonising and managing metadata across government departments, resulting in better contextualised training data for AI models – which in turn will help to improve public services.
People and skills
The recent step-change in the capabilities of AI created a steep learning curve for us all. Recent discussions with key stakeholders in the UK government have articulated a justifiable concern in the readiness of users to trust the outputs of generative AI and to assume they understand how the technology works. It’s unsurprising that these concerns around AI literacy are slowing government uptake of the technology, what with the evident risks. However, this is not an insurmountable challenge, and it can be tackled in various ways including through training programmes and integrating guardrails into AI implementations. Indeed, as my colleague (and former GDS Deputy Director) Jessica McEvoy argues in this blog post, civil service leaders already have many of the skills they need to oversee the safe use of AI.
As in other sectors, it’s likely that AI will take on a range of tasks that civil servants used to do, prompting fears of job losses. AI is unlikely to reduce job numbers in the long run because, historically, new technologies tend to create as many roles as they replace, though often in different areas. As AI automates repetitive tasks, it frees up civil servants for more complex and creative roles that require human judgment, empathy, and problem-solving. Not only that, but the management of AI itself is likely to generate a range of new roles. Government will need people with AI-specific expertise in data quality management, security and privacy protection, system auditing, bias detection and mitigation, and oversight and governance. As AI technologies mature and AI literacy grows, civil servants will be quick judges of which use cases are suited to the application of AI and which are not. Consultancies can help civil servants on this learning curve. We recently supported a team at the Department for Work and Pensions to grow its capabilities in Machine Learning Operations (MLOps) so that it can further enhance its services for citizens.
I’m not underestimating the scale of the challenge ahead for the UK government in safely harnessing AI to improve public services – and taking citizens along with them on that journey. However, as a former leader of the Government Digital Service myself, I know that they’re equal to the challenge. A key takeaway from recent discussions with government has been the ambition, pragmatism and judicious caution expressed. From a standing start in 2011, GDS led the digital transformation of government and created digital public services that are truly world leading. With the same ambition, rigour and clarity of purpose, there’s no reason why the government can’t address the blockers and provide an exemplar of AI adoption for the public good.
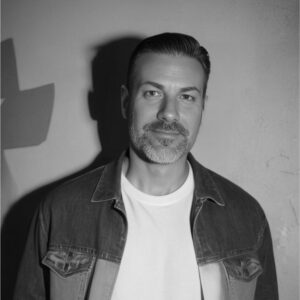
Stephen Foreshew-Cain
Stephen Foreshew-Cain is CEO of Scott Logic and former Executive Director of the UK Government Digital Service.