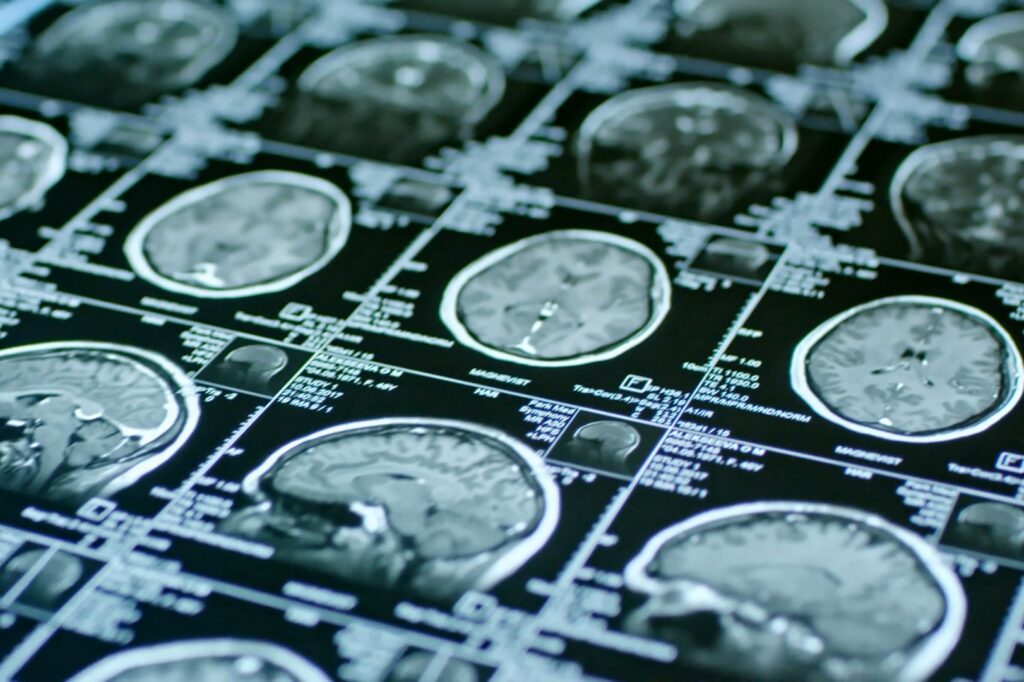
AI is set to transform the pharmaceutical and healthcare industry in the coming years by improving decision making, automating repeatable processes, and speeding up vital research. Interest in the technology is undoubtedly growing, with a GlobalData survey of 198 pharma companies voting that AI will be “the most disruptive technology across the pharmaceutical industry”.
Particularly, AI can reduce the amount of time spent analysing and processing data. The majority of scientists still spend almost half their time ‘wrangling’ data, distracting them from revealing valuable insights. AI is especially useful for enabling the evolution of precision medicine and sequencing genomics, where there is a huge volume of scientific literature and real-world patient data, analysis of which could lead to valuable insights.
AI approaches such as text mining and natural language processing (NLP) have been especially beneficial in the search for new treatments for rare diseases. A good example is a recent collaborative project between Elsevier and the Sinergia Consortium (DMG/DIPG Center at University Children’s Hospital Zurich; ETH Zurich; the Centre for Molecular Medicine Norway (NCMM)), and the Open Pediatric Brain Tumor Atlas project.
Building an AI disease model
The project seeks to develop an AI disease model for diffuse intrinsic pontine glioma (DIPG), which is a particularly aggressive form of brain tumour that targets children. Currently, there are no effective treatments for DIPG, and the typical life expectancy for a child after diagnosis is eight to 11 months.
Disease models aid understanding of how a particular disease develops (e.g., risk factors or triggers) and enable improved testing of treatment approaches. The DIPG project aims to develop a model that will use NLP and machine learning to identify FDA-approved drugs that could be candidates for repurposing. The initial models were built using Elsevier’s Biology Knowledge Graph and complementary software, which analysed ‘OMICs data’ (genomics, proteomics, metabolomics, metagenomics and transcriptomics) from real-world patients with DIPG.
Discovering how tumours mutate
By analysing patient OMICs data, the team was able to examine protein activity and identify the most active genes in DIPG tumours. They then mapped most active proteins against the cancer hallmarks models, proposed by Hanahan & Weinberg and developed by Elsevier scientists, to understand the driver mutations that are responsible for tumour growth. The project also used text mining of existing scientific literature to the expanded cancer hallmark collection and to link mutations to the most active pathways. This helped to understand the disease mechanism more and find better drugs.
To train the AI disease model further, the Children’s Hospital of Philadelphia developed the cloud-based platform Cavatica, which includes DIPG data for more than 30 patients – indicating the genetic mutations and gene expression. The scale of the data for each patient is immense, containing 3,000 and 5,000 mutations and between 2,000 and 3,000 additional genetic mutations in the cancer patients. Since this is beyond human capability to sift through manually, AI was able to help analyse the data and discover two major cancer hallmarks that were active in all 30 DIPG patients – which could help to find new effective treatments.
Using the disease model to find potential drug candidates
Once the algorithm had uncovered which proteins cause tumours to form, the next step was to uncover drugs that repress the activity of these proteins. 637 drugs were uncovered initially, but these drugs were then narrowed down using NLP (applying Elsevier’s AI deep reading and text mining) to look for the ones that specifically inhibited a mutation called TP53.
Finally, this model was leveraged to find FDA-approved drugs which inhibit the disease mechanism, since using pre-approved drugs means new treatments can be brought to patients faster. To find the ten drugs with the most potential for experimental validation, further drug ranking scores were developed. One key concern when reviewing drugs was toxicity, especially for children as DIPG patients are commonly between five and ten years old. Data from PharmaPendium was used to obtain toxicity profiles of found drugs.
Outcomes and the future of AI
The AI disease model for DIPG currently has 19 pathways, each of which is dedicated to cell type, biological processes, cell differentiation state and disease state. The project uncovered 212 drugs that reverse protein activity in disease state, and 25% of these drugs can inhibit mutant TP53 by four different mechanisms.
This is just one example of how AI can advance drug discovery and treat rare diseases, but this work can also provide a model to apply to other disease areas. When this model is eventually scaled, AI will allow further precision medicine approaches for a variety of rare diseases.
AI will undoubtedly play a critical role in the future of life sciences R&D. However, the reality today is that life sciences is only just scratching the surface of what AI, alongside human ingenuity, can do. As more use cases such as this project are brought into the spotlight, organisations will realise the benefits even faster – helping to advance our understanding of disease and bring vital therapeutics to patients sooner.
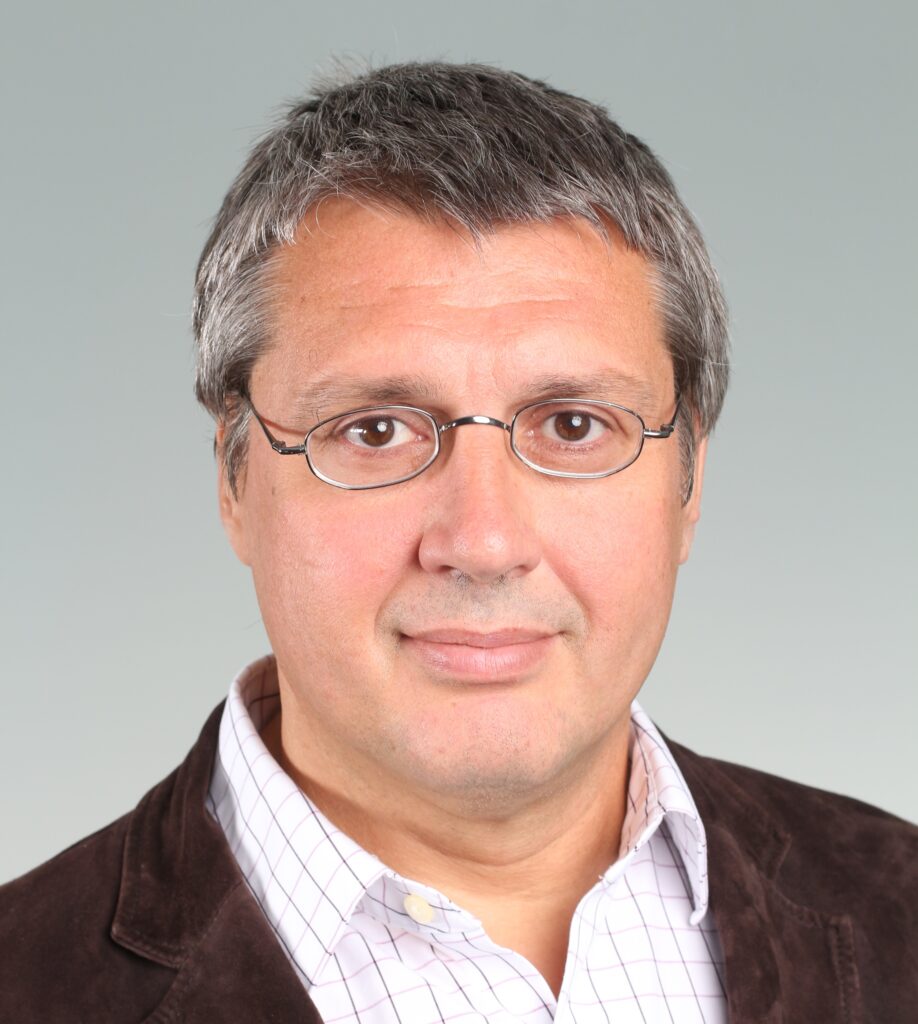
Dr. Anton Yuryev is the Professional Services Director at Elsevier, and focuses on developing bioinformatics solutions to meet customer needs through consulting, custom software development and custom analytics. His current research focuses on studying topological and evolutionary properties of biological networks and developing algorithms and workflows for pathway reconstruction. methods.