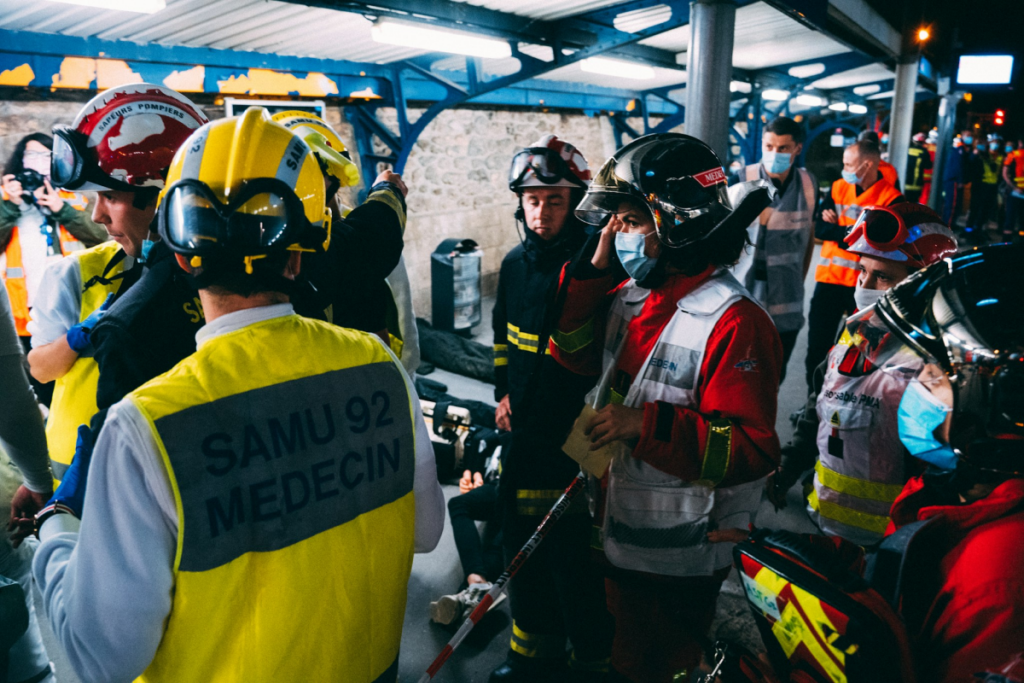
The COVID-19 pandemic challenged every government’s readiness – or unreadiness – to respond to a major disaster. Much was made of contact tracing: the need for public bodies to quickly and efficiently identify exactly where cases had originated and who had been in contact with ‘patient zero’. If public health officials knew who was close to an individual with Covid, they could tell the right people to self-isolate, and fast.
Contact tracing happened, but initially relied on patchy data. In the UK, it later required the voluntary involvement of the entire population via the NHS COVID-19 app. As a result, without access to the right data, the ability of the app to accurately track exactly who had been in contact with the virus was stretched.
This challenge – needing accurate, real-time data to respond to crises – isn’t unique to governments. Many organisations, both public and private, don’t easily have access to the data they need to respond to a crisis, and when they do have the data, they often aren’t measuring its quality to identify any quality problems. So, in an emergency situation, when data is needed in real-time, it is often cobbled together – ‘any data is better than no data’, exacerbating the issue of poor data quality.
Robust data management is essential to navigate disruptions and emergencies. Whether a pandemic or a natural disaster blocking supply routes, companies need real-time, accurate data to make swift, informed decisions. For example, a medical company facing sudden supply chain disruptions due to severe weather needs to know where inventory is located, which routes are accessible, and what products are available from alternative suppliers. If one route or supplier becomes unavailable, they can quickly pivot to another. This kind of data can mean the difference between delivering life-saving medicine and not getting it to the patients in need.
Whatever the problem, organisations need to have the right data management strategy to build the best possible disaster response. There’s no time to fix it in the moment. Only a firm data foundation built in the good times can give organisations the confidence that decisions in the moment, are based on the best information available.
Poor data has an outsized impact in disaster response
The stakes couldn’t be higher than in a disaster response situation. For example, in a severe weather situation, low-quality data can lead to misallocated resources, like food, medical equipment and other life-saving essentials. Organisations often don’t have the data assets they need at hand to act on. So they rush to source and merge the data they think they need, compromising quality and skipping essential management principles. This can result in poorly deployed teams, mis-prioritised or wrongly distributed life-saving medicine, food or transport. And this can mean the difference between people being quickly helped, and people waiting weeks for aid. That’s a serious problem in the immediate moment – and in the long-term, it erodes public trust.
No matter the organisation – and this includes healthcare providers, logistics and delivery companies, pharmaceutical organisations and so on – the foundations for response need to be strongly constructed. Using spreadsheets and non-digital, manual mechanisms for reporting purposes increases the risk responding organisations will feed delayed or inaccurate data into their prediction models that are used to make policy. And when the fire’s burning or a disease is spreading, poorly informed policy has very real effects on people’s lives.
Good data optimises essential tasks
To ensure success, let’s go back to the example of contact tracing during a pandemic. If the right data has been consistently collected and efficiently managed, organisations can leverage automated verification and enrichment of address data, improving the speed and accuracy of the programme.
Accurate contact data might seem obvious, but the all-pervasive nature of data means the full effect of its quality across a disaster response programme may not be immediately clear. With accurate data, you can be sure you’re not wasting time and risking an ineffective response by correcting the basics—names, addresses, phone numbers, and so on.
It allows you to build a more granular, detail-orientated response policy that’s easy to scale. If you don’t verify data accuracy at the point of entry and then verify it on a regular basis (or get someone to do that for you), it will inevitably become a problem that requires everyone’s attention.
How data management makes major campaigns work
We can see how good data management supports good outcomes on a major public scale by looking at a case study. One of our customers, a large healthcare organisation in the USA, serves over 440,000 people in 32 cities. Its mission is to support healthier communities with exceptional, world-class healthcare in a regional healthcare setting. It wanted to use advancing technology to make better healthcare decisions, increase patient appointments, and improve the patient experience.
Using cloud data integration and management to feed data from multiple systems into Microsoft Dynamics, it was able to maintain e-messaging standards and increase patient appointments by 300%. In short, the right tools make data handling much easier – and it’s not hard to see how improving population health via trusted, clean data can help in dealing with a post-crisis landscape.
The role of AI and why the right approach to data is needed
There’s a lot of excitement about how AI models can improve everything from filmmaking to investment banking, and disaster relief is no exception. In a fast-evolving crisis, with data pouring in from across a region or nation, AI seems to promise superhuman processing and decision-making.
Consider a product recall: a manufacturer must quickly trace affected items across a complex supply chain. With high-quality, accessible data, AI can pinpoint the exact locations and quantities of defective products, allowing rapid notifications to retailers and consumers. But without accurate, unified data, even the best AI models may struggle, leading to delays that could, in the worst cases, significantly impact the safety of customers.
Before harnessing AI to improve disaster response, organisations need the right data foundations in place. Our research shows one-third of senior data leaders lack a complete view and full understanding of their organisation’s information. Without this view, it’s near impossible to implement a fully formed AI capability. This lack of readiness creates a “disaster waiting to happen”, as inadequate data management could lead to significant failures when AI is applied.
Organisations need to prioritise data management principles to ensure data is holistic, accurate, up-to-date, accessible and protected. This includes investing in simplified data management technology to alleviate technical debt and foster innovation. A unified platform approach integrates diverse data sets so organisations can accelerate the delivery of data systems and empower frontline users with data at their fingertips, enabling data-led decision-making.
Disaster response is one of the most crucial and challenging responsibilities of government. And businesses can also play a significant role in tackling challenges head-on using a similar preventative approach. If an organisation is equipped with all the relevant knowledge on how their data will perform when leveraged by AI, they could reduce their level of risks if facing issues such as, product recalls, medicine shortages, supply chain issues or even natural disasters. Thankfully, we live in an era when large-scale data modelling can transform how resources are prioritised and deployed. Acting now, with the right data management backbone in place, both government agencies and organisations can harness that potential and develop the kind of policies and plans that save lives and protect communities.
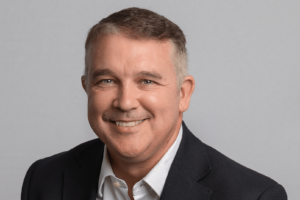
Greg Hanson
Greg Hanson is GVP EMEA North at Informatica.