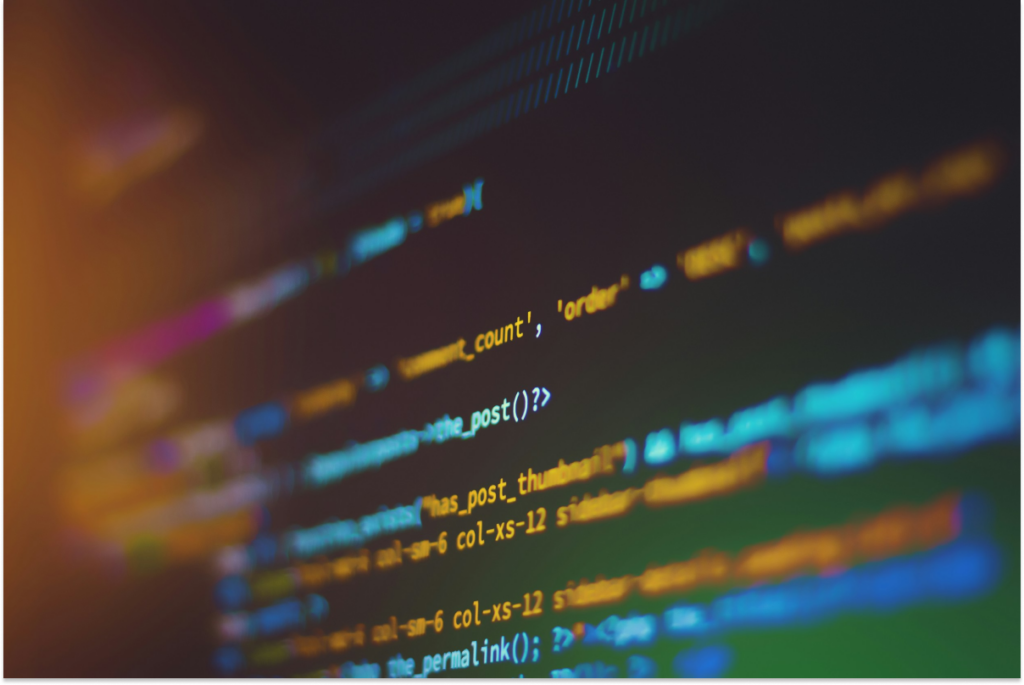
Access to finance in underserved demographics remains a problem. While there has been a push to improve access to credit globally, gaps in financial inclusion persist, disproportionately affecting women and individuals under 25. This is particularly prevalent in emerging markets. For example, in West Africa women make up only 27% of contract borrowers, and, in Kenya, only 0.4% of all 20- to 24-year-olds received formal loans of over $200 in 2023.
These limitations impact the lives of thousands of people. Without a strong credit score they are unable to secure housing, financing, or even mobile phone plans. Expanding financial inclusion allows more people, especially underserved groups, access to capital for these and other purposes. It also helps them launch and grow businesses, creating jobs and boosting the local economy.
While there are many approaches to achieving fair and widespread access to finance, alternative data and artificial intelligence (AI) offer powerful tools to overcome existing barriers and help close the financial gap. Read on to learn more.
What is alternative data?
Traditional credit scoring models typically rely on conventional data obtained from regular consumer bank transactions or prior payment records, potentially excluding millions of people from accessing loans. Whereas alternative data includes information on telecom and utility usage, previous mobile or small loans, data on related parties, and self-reported data — all of which ideally obtainable through credit bureaus.
More specifically, mobile transaction data can inform banks and lenders about an individual’s income and cash flow, acting as indicators of their ability to make repayments. Similarly, social media activity, including comments on posts and instant messenger interactions, can detect fraudulent behaviours, helping to build risk profiles for individuals. Credit scoring models may also utilise satellite images, for example, of property or agricultural land, to assess asset value.
By incorporating alternative sources of data, financial institutions can create more comprehensive and accurate credit profiles for underserved groups – even those with limited or no traditional credit history.
The role of AI in credit scoring
Effectively using large volumes of alternative data requires robust systems, enabled by AI and machine learning models that can efficiently analyse and derive insights from both structured and unstructured data sources for credit scoring. Financial institutions need to establish secure model management frameworks to oversee, control, and deploy algorithms built on alternative data, which can identify patterns and trends that would otherwise go undetected if left to the human eye.
AI-powered tools can also provide personalised financial advice and education, helping individuals – especially young people – make informed decisions about their finances. Another useful feature of AI and alternative data is personalising financial products for individual customer needs, for example personalised loans with flexible repayment terms, or insurance policies that match specific needs.
It’s important to remember that AI technology can’t fully replace the work of people but that it can be used to enhance processes and make them more efficient.
Balancing AI and human insight
AI is a powerful tool, but its effectiveness depends heavily on how it’s used. One challenge with using AI for credit scoring is the risk of bias, which requires human oversight to catch these biases. Models trained on historical data may replicate existing biases present in that data and favour directly or indirectly certain groups based on factors like race, gender, or socioeconomic status. This can lead to discriminatory outcomes and may ultimately undermine efforts to promote financial inclusion.
Data privacy and security are paramount in this process. AI-powered credit scoring relies on a wide range of data, including often sensitive information, and so protecting this data from breaches and misuse is crucial. Similarly, the accuracy of the data used to train AI models is essential. Poor data quality can again lead to inaccurate and biased predictions.
Ultimately, it’s a pick-and-mix approach. For best results, credit modelers should aim to cherry-pick the best bits of AI and machine learning tools, combining them with a human approach. As much as AI is very useful for automation, it still needs people to interpret the models to ensure accurate and non-biased results.
Conclusion
Financial inclusion is crucial for a resilient economy. In a climate with rising inflation and global economic uncertainty, countries need strong foundations to protect their economies. Alternative data is a powerful tool to support financial inclusion globally, but ensuring its secure collection, responsible use, and authorised sharing is key. By combining advanced AI, machine learning models, and clear regulatory frameworks, financial institutions can unlock the potential of this data to expand access to finance. This approach fosters greater local economic participation, driving sustainable and inclusive growth that benefits entire regions and nations.
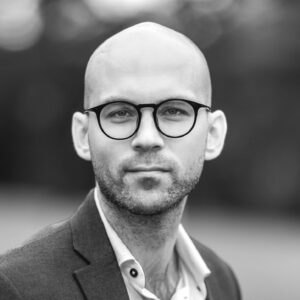